Data Science Services
Data Science Services
IQSS Data Science Services is pleased to assist researchers in the Harvard community working on publication research in overcoming obstacles at every stage of the research process.
Advisory Services
Consulting
We provide short-term support (up to 3 hours per publication) in the form of advice.
DSS Service Details
Collaboration
We provide extended support over the lifecycle of a research project by embedding a data science specialist in your research team.
DSS Service Details
Analytical Tool Building
We can develop R code to implement your new statistical method or to automate part of your analysis pipeline.
DSS Service Details
Training Services
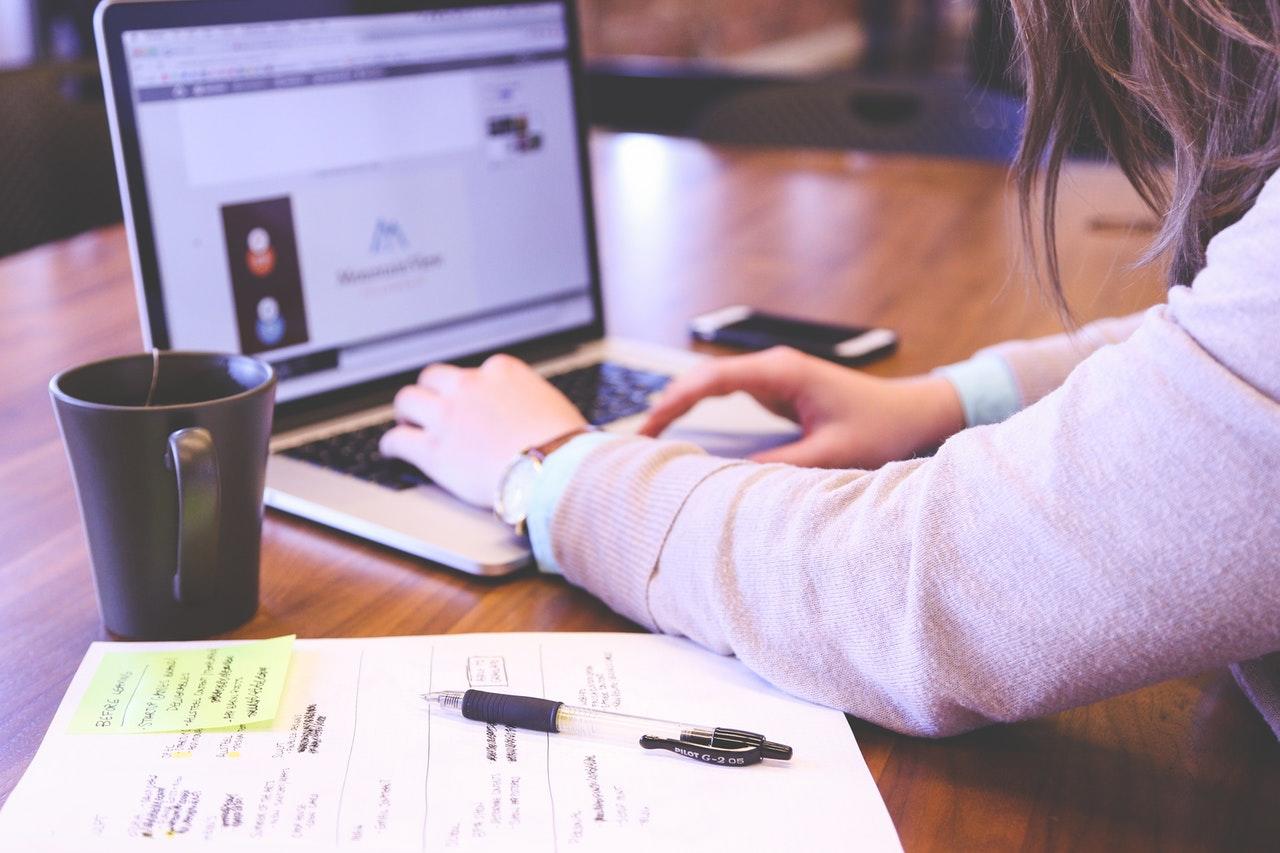
Related Resources
We here provide a list of additional data science resources across IQSS and Harvard University.
Service Details
Impact
Performance Metrics
We aim to build and coordinate a broader community of Harvard computing/data science consultants along with other goals to improve data research support.
Learn more about DSS performance metrics
We are always very interested to hear about research that has been impacted by Data Science Services.
Learn more about research output
Data Science Services Team
How to Get Help
If you want to request a consultation or have questions about our other services, we are here to help.